Machine-learning-accelerated design of high-performance platinum intermetallic nanoparticle fuel cell catalysts
Posting date on solidfuturism: January 25th 2024
Published date: January 10th 2024
Authors: Peng Yin, Xiangfu Niu, Shuo-Bin Li, Kai Chen, Xi Zhang, Ming Zuo, Liang Zhang & Hai-Wei Liang
DOI: https://doi.org/10.1038/s41467-023-44674-1
Abstract composer: Seyed Amirhosein Mirsadri
Have you ever heared about fuel cells, the most promissing and innovative green source of energy in recent years? well, there is some problems in reduction of oxygens in such cells, and the answer was using a proper platinum based catalyst!. But the platinum usage is a global concerning problems these days for its high cost and heavy use, and this is why that this team of research have established research on finding how to make these catalyst more efficient with lesser impact with alloying it with more metals with retained or more efficiency. They found out Pt-Co nanoparticles are good enough for the oxygen reduction reacions (ORR) and also it was investigated that the size and the atom distribution of the nanoparticles are the most disscussable factors that have direct impact on ORR. The research undergoes alloying Pt-Co atmic composition with 16 new different atoms: Na, Mg, Sc, Ti, Mn, Fe, Ni, Cu, Ga, Ge, In, Sn, Sb, Te, Pb and Bi to make Pt-Co/M (M stand for 16 mentioned atoms). As we all know reaching this amount of synthesized nanocomposite is so costly and will take so much time to investigate.
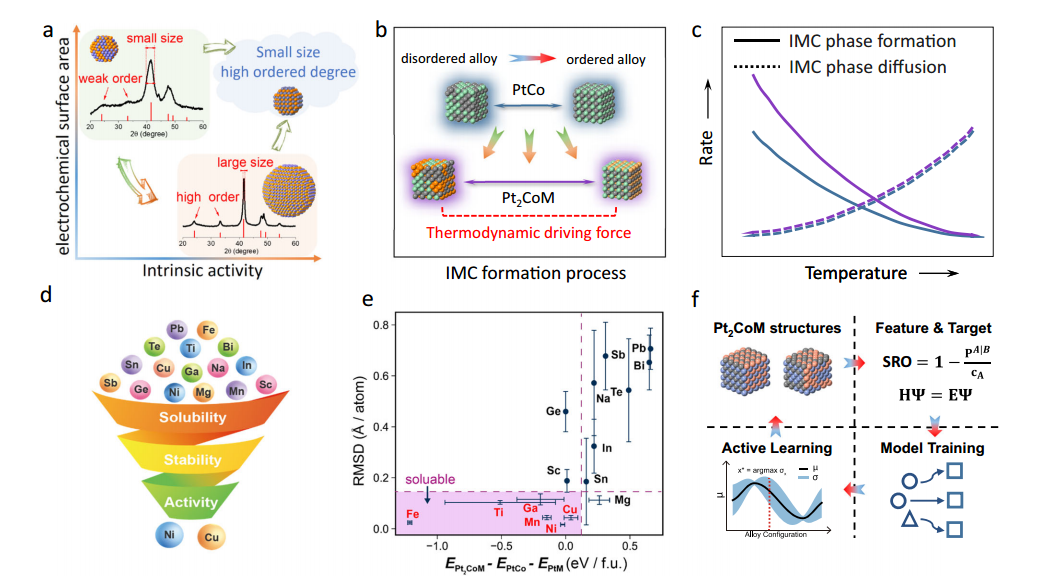
Therefore the research can be first predicted by the statistical model calculations or by automated machine learning evaluations!. The automated machine learning process, first needs to define a proper input and output but how we chose a proper one? It was investigated that: 1) the size of the nanocomposite will be in opposite relations with the ORR and also in direct proportion with the conducting resistance. 2) The distribution of the atoms and layers in the mettalic alloy is very important, meaning that when it is more ordered or well arranged the efficiency will be increased more. Therefore, we obtain what we should search as an input for machine learning, how the atom is arranged together as an input and eventually what energy does it need to form a ordered alloy as an output. In fact, there is a need for thermodynamically and kinetic energy to make a disordered alloy to a ordered one which mostly it is done by applying temperature to it. To that end, rather than we apply higher degrees of temperatures, we could reach that ordered alloy similarely by adding third metallic atom in the structure that helps to regulating the structure evenly. The calculations for predictions was performed by density functional theory (DFT) and warren-cowley short range order (SRO) in a Gaussian Process Regression (GPR) for machine learning uncertainty measurements. In SRO and DFT models, two values were defined: apt-(CO/M)=-1/3 for the ordered alloy with -1/3 unit of energy needed to reach that point and apt-(CO/M)= 0 for the disordered strucure with 0 energy needed to alter the structure.
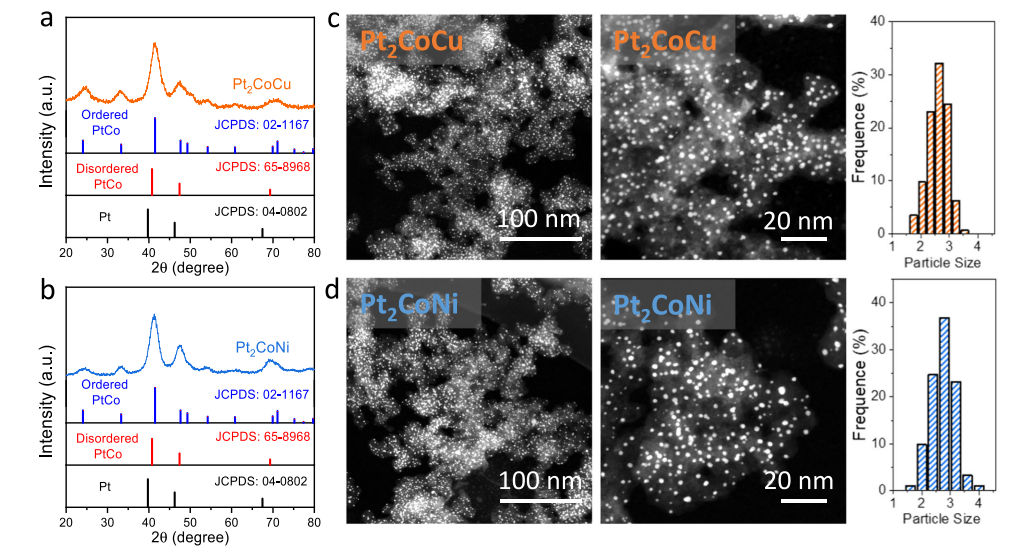
After introducing the layer distribution in DFT method with 3*3*3 and 4*4*1 (Monkhorst-Pack k-point) models different possible absorbing energies will be obtained for OH and O in different structures. The solubility test for ordering the structure was performed under monte-carlo algorithm in Warren-Cowley short range calculations. This algorithm generates fast structures for given possible values with the mentioned forulas: apt-(CO/M)=-1/3 for ordered and apt-(CO/M)= 0 for disordered alloys in the range number of -1/6, -1/3 , -2/6, 0, 1/6, 2/3, 2/6 and 3/6. After defining these parameters, the values must be explained for the machine to search for look up of data to learn from the given data and future data to automatically find and predict best structure with selected metals with proper conditions. The machine learns and analyses the data through built-in mentioned formulas (DFT and warren-cowley).
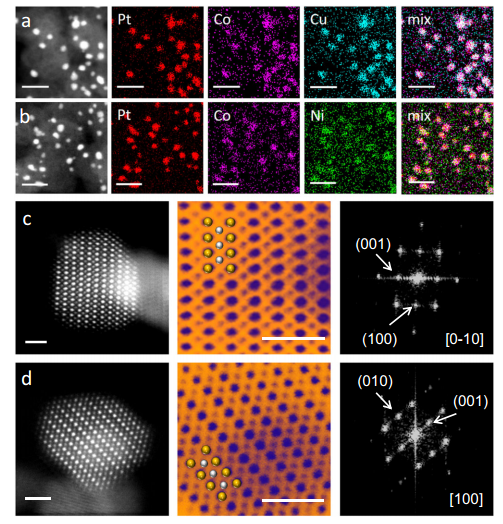
The machine predicted that alloying the Pt-Co with Ni and Cu will result significant effectiveness in Oxygen reduction. Therefore experiments and synthesis were performed under such conditions and showed very good result as expected. The wet successfull synthesis was characterized via XRD, SEM, EDX, CV (cyclic voltametery) , LSV (linear sweap voltametery), HAADF-STEM and RDE rotating disk electrode for ORR analysis. The structures was defined as a core-shell nanoparticles with order of Pt as shell and Pt-Co/M as core to prevent Pt poisoning during electro analysis. The catalyst were immobillized on the surface of the electrode with naphione and alchol and ultrasonic spray.